Comparación de Modelos de Aprendizaje para la Identificación de Pacientes Depresivos por Medio de Actividad Motora
DOI:
https://doi.org/10.17488/RMIB.46.1.1487Palabras clave:
actividad motora, análisis de datos, aprendizaje automático, depresión, minería de datosResumen
El presente estudio tiene como objetivo evaluar diversos algoritmos de clasificación de datos pertenecientes a sujetos diagnosticados con depresión y sujetos no depresivos. Para ello, se analizaron los datos obtenidos del dataset "depresjon" propuesto por Garcia-Ceja, E., et al, el cual se compone de la actividad motora captada por el dispositivo Actiwatch (Cambridge Neurotechnology Ltd, England, model AW4). Mediante distintos modelos de aprendizaje automático se realizaron predicciones incluyendo datos sintéticos. Posteriormente, se compararon métricas como especificidad, sensibilidad y precisión. Los resultados muestran las mejores características de los datos, así como el mejor modelo de aprendizaje automático (mediante modelo de ensamble) para realizar la clasificación de posibles episodios depresivos en la actividad durante la tarde y la noche, con una precisión del 96.6 %, una sensibilidad del 100 % y una especificidad del 93.33 %.
Descargas
Citas
M. E. Morris and A. Aguilera, “Mobile, social, and wearable computing and the evolution of psychological practice,” Prof. Psychol. Res. Pract., vol. 43, no. 6, pp. 622-626, 2012, doi: https://doi.org/10.1037/a0029041
C. A. Woody et al., “A systematic review and meta-regression of the prevalence and incidence of perinatal depression,” J. Affect Disord, vol. 219, pp. 86-92, 2017, doi: https://doi.org/10.1016/j.jad.2017.05.003
J. G. Rodríguez-Ruiz et al., “Comparison of night, day and 24 h motor activity data for the classification of depressive episodes,” Diagnostics (Basel), vol. 10, no. 3, 2020, art. no. 162, doi: https://doi.org/10.3390/diagnostics10030162
I. V. Vahia and D. D. Sewell, “Late-life depression: a role for accelerometer technology in diagnosis and management,” Amer. J. Psychiatry, vol. 173, no. 8, pp. 763-768, 2016, doi: https://doi.org/10.1176/appi.ajp.2015.15081000
A. Abd-Alrazaq et al., “Wearable artificial intelligence for anxiety and depression: scoping review,” J. Med. Internet Res., vol. 25, 2023, art. no. e42672, doi: https://doi.org/10.2196/42672
E. Garcia-Ceja et al., “Depresjon: a motor activity database of depression episodes in unipolar and bipolar patients,” in Proc. 9th ACM Multimedia Syst. Conf., Amsterdam, Netherlands , 2018, pp. 472-477, doi: https://doi.org/10.1145/3204949.3208125
A. Massaro et al., “Decisional support system with artificial intelligence oriented on health prediction using a wearable device and big data,” in 2020 IEEE Int. Workshop Metrol. Ind. 4.0 & IoT, Roma, Italy, 2020, pp. 718-723, doi: https://doi.org/10.1109/MetroInd4.0IoT48571.2020.9138258
D. Wang, J. Weng, Y. Zou, and K. Wu, “EmoTracer: a wearable physiological and psychological monitoring system with multi-modal sensors,” in Adjunct Proceedings of the 2022 ACM International Joint Conference on Pervasive and Ubiquitous Computing and the 2022 ACM International Symposium on Wearable Computers, New York, NY, USA, 2022, pp. 444-449, doi: https://doi.org/10.1145/3544793.3560409
P. M. Singh and P. Sathidevi, “Design and implementation of a machine learning-based technique to detect unipolar and bipolar depression using motor activity data,” in Smart Trends in Computing and Communications, vol. 286, 2021, pp. 99-107, doi: https://doi.org/10.1007/978-981-16-4016-2_10
C. Iribarne, V. Renner, C. Pérez, and D. L. de Guevara, “Trastornos del Ánimo y Demencia. Aspectos clínicos y estudios complementarios en el diagnóstico diferencial,” Rev. Méd. Clín. Las Condes, vol. 31, no. 2, pp. 150-162, 2020, doi: https://doi.org/10.1016/j.rmclc.2020.02.001
G. Jackson-Koku, “Beck Depression Inventory,” Occup. Med., vol. 66, no. 2, pp. 174-175, 2016, doi: https://doi.org/10.1093/occmed/kqv087
J. Morrison. DSM-5® Guía para el diagnóstico clínico. (2015). [Online]. Available: https://cdn.website-editor.net/50c6037605bc4d1e9286f706427108e6/files/uploaded/DSM-5%2520guia%2520para%2520el%2520diagnstico%2520clinico%2520-%2520James%2520Morrison.pdf.
J. Davidson et al., “The Montgomery‐Åsberg Depression Scale: reliability and validity,” Acta Psychiatr. Scand., vol. 73, no. 5, pp. 544-548,1986, doi: https://doi.org/10.1111/j.1600-0447.1986.tb02723.x
World Health Organization, 2015, “International statistical classification of diseases and related health problems,” distributed by World Health Organization, https://iris.who.int/handle/10665/246208.
S. Saeb et al., “The relationship between clinical, momentary, and sensor-based assessment of depression,” in 2015 9th International Conference on Pervasive Computing Technologies for Healthcare (PervasiveHealth), Istanbul, Turquía, 2015, doi: https://doi.org/10.4108/icst.pervasivehealth.2015.259034
Z. Y. Wee et al., “Actigraphy studies and clinical and biobehavioural correlates in schizophrenia: a systematic review,” J. Neural. Transm., vol. 126, no. 5, pp. 531-558, 2019, doi: https://doi.org/10.1007/s00702-019-01993-2
J. O. Berle et al., “Actigraphic registration of motor activity reveals a more structured behavioural pattern in schizophrenia than in major depression,” BMC Res. Notes, vol. 3, no. 1, 2010, art. no. 149, doi: https://doi.org/10.1186/1756-0500-3-149
R. Ramakrishnan et al., “Accelerometer measured physical activity and the incidence of cardiovascular disease: Evidence from the UK Biobank cohort study,” PLoS Med., vol. 18, no. 1, 2021, art. no. e1003487, doi: https://doi.org/10.1371/journal.pmed.1003487
B. Helgadóttir, Y. Forsell, and Ö. Ekblom, “Physical activity patterns of people affected by depressive and anxiety disorders as measured by accelerometers: a cross-sectional study,” PLoS One, vol. 10, no. 1, 2015, art. no. e0115894, doi: https://doi.org/10.1371/journal.pone.0115894
A. C. Cote et al., “Evaluation of wearable technology in dementia: a systematic review and meta-analysis,” Front. Med., vol. 7, 2021, art. no. 501104, doi: https://doi.org/10.3389/fmed.2020.501104
V. Trevino and F. Falciani, “GALGO: an R package for multivariate variable selection using genetic algorithms,” Bioinformatics, vol. 22, no. 9, pp. 1154-1156, 2006, doi: https://doi.org/10.1093/bioinformatics/btl074
W. S. Noble, “What is a support vector machine?,” Nat. Biotechnol., vol. 24, no. 12, pp. 1565-1567, 2006, doi: https://doi.org/10.1038/nbt1206-1565
B. E. Boser, I. M. Guyon, and V. N. Vapnik, “A training algorithm for optimal margin classifiers,” in Proc. COLT92 5th. Annu. Workshop Comput. Learn. Theory, Pittsburgh, PA, USA, 1992, pp. 144-152, doi: https://doi.org/10.1145/130385.130401
A. Krogh, “What are artificial neural networks?,” Nat. Biotechnol., vol. 26, no. 2, pp. 195-1977, 2008, doi: https://doi.org/10.1038/nbt1386
G. Guo et al., “KNN model-based approach in classification,” in On The Move to Meaningful Internet Systems 2003: CoopIS, DOA, and ODBASE, Sicily, Italy, vol. 2888, 2003, pp. 986-996, doi: https://doi.org/10.1007/978-3-540-39964-3_62
B. Mahesh et al., “Machine learning algorithms-a review,” Int. J. Sci. Res., vol. 9, no. 1, pp. 381-386, 2020, doi: https://doi.org/10.21275/ART20203995
M. Gashler, C. Giraud-Carrier, and T. Martinez, “Decision tree ensemble: Small heterogeneous is better than large homogeneous,” in 2008 7th Int. Conf. Mach. Learn. Appl., San Diego, CA, USA, 2008, pp. 900-905, doi: https://doi.org/10.1109/ICMLA.2008.154
A. B. Shaik and S. Srinivasan, “A brief survey on random forest ensembles in classification model,” in Proc. 2018 Int. Conf. Innov. Comput. Commun., vol. 56, Nov. 2018, pp. 253-260, doi: https://doi.org/10.1007/978-981-13-2354-6_27
L. Pietruczuk, L. Rutkowski, M. Jaworski, and P. Duda, “How to adjust an ensemble size in stream data mining?,” Inf. Sci., vol. 381, pp. 46-54, 2017, doi: https://doi.org/10.1016/j.ins.2016.10.028
S. Chetlur et al., Dec. 2014, “ccuDNN: Efficient primitives for deep learning,” 2014, arXiv:1410.0759, doi: https://doi.org/10.48550/arXiv.1410.0759
A. C. Davison and D. V. Hinkley, Bootstrap methods and their application (Cambridge Series in Statistical and Probabilistic Mathematics). New York, NY, USA: Cambridge University press, 1997, doi: https://doi.org/10.1017/CBO9780511802843
Y. Xie et al., “Stacking ensemble learning models for daily runoff prediction using 1D and 2D CNNs,” Expert Syst. Appl., vol. 217, 2023, art. no. 119469, doi: https://doi.org/10.1016/j.eswa.2022.119469
I. M. Lin et al., “Prefrontal Lobe and Posterior Cingulate Cortex Activations in Patients with Major Depressive Disorder by Using Standardized Weighted Low-Resolution Electromagnetic Tomography,” J. Pers. Med., vol. 11, no. 11, 2021, art. no. 1054, doi: https://doi.org/10.3390/jpm11111054
S. Wang, F. Leri and S. J. Rizvi, “Anhedonia as a central factor in depression: Neural mechanisms revealed from preclinical to clinical evidence,” Prog. Neuro-Psychopharmacol. Biol. Psychiatry vol. 110, 2021, art. no. 110289, doi: https://doi.org/10.1016/j.pnpbp.2021.110289
J. Chen, A. Zeb, Y. A. Nanehkaran, and D. Zhang, “Stacking ensemble model of deep learning for plant disease recognition,” J. Ambient Intell. Human. Comput., vol. 14, pp. 12359-12372, 2023, doi: https://doi.org/10.1007/s12652-022-04334-6
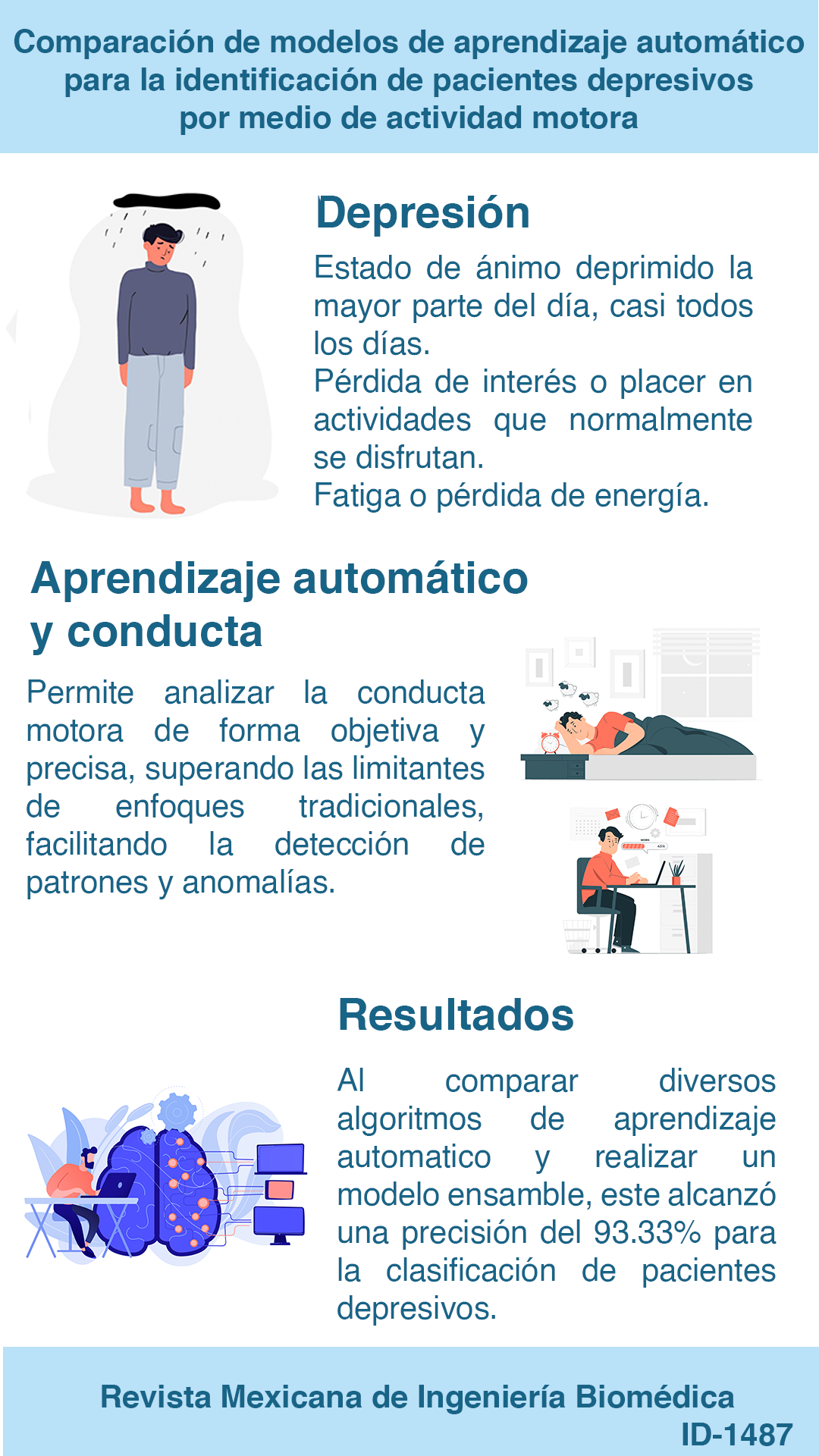
Descargas
Publicado
Cómo citar
Número
Sección
Licencia
Derechos de autor 2025 Revista Mexicana de Ingenieria Biomedica

Esta obra está bajo una licencia internacional Creative Commons Atribución-NoComercial 4.0.
Una vez que el artículo es aceptado para su publicación en la RMIB, se les solicitará al autor principal o de correspondencia que revisen y firman las cartas de cesión de derechos correspondientes para llevar a cabo la autorización para la publicación del artículo. En dicho documento se autoriza a la RMIB a publicar, en cualquier medio sin limitaciones y sin ningún costo. Los autores pueden reutilizar partes del artículo en otros documentos y reproducir parte o la totalidad para su uso personal siempre que se haga referencia bibliográfica al RMIB. No obstante, todo tipo de publicación fuera de las publicaciones académicas del autor correspondiente o para otro tipo de trabajos derivados y publicados necesitaran de un permiso escrito de la RMIB.