Aplicación de Redes Neuronales Artificiales para la Clasificación de Actividades de la Vida Diaria en Sujetos con Enfermedad de Párkinson
DOI:
https://doi.org/10.17488/RMIB.44.4.9Palabras clave:
enfermedad de Párkinson, PCA, redes neuronales artificialesResumen
El presente trabajo es un seguimiento a la propuesta para la contribución con especialistas en la salud para enriquecer los sistemas de seguimiento y apoyo en pacientes con Enfermedad de Párkinson a través de la clasificación de actividades de la vida diaria (AVDs) utilizando Redes Neuronales Artificiales programadas en lenguaje Python. El método propuesto de aprendizaje supervisado permitió la clasificación de 6 AVDs mediante 22 señales procedentes de haber aplicado Análisis de Componentes Principales; conformando la base de datos utilizada para entrenar un Perceptrón Multicapa, logrando un acercamiento a la clasificación con el 93% de medida F1-score. El presente estudio demuestra la versatilidad de las RNA basadas en MLP combinadas con la técnica de PCA, pues incluso en una base de datos desbalanceada como la utilizada permite alcanzar excelentes valores en la medida F1-score. El uso de Inteligencia Artificial y otras herramientas aplicadas en este trabajo pueden eventualmente ayudar a especialistas a desempeñar una evaluación más certera en el monitoreo de la rehabilitación en pacientes con enfermedad de Párkinson mejorando los registros y así evitar subjetividad en la interpretación de los resultados del tratamiento.
Descargas
Citas
A.-E. Gómez Ayala, “Enfermedad de Parkinson,” Offarm, vol. 26, no. 5, pp. 70–78, 2007. [En línea]. Recuperado de: https://www.elsevier.es/es-revista-offarm-4-articulo-enfermedad-parkinson-13102417
R. Martínez-Fernández, C. Gasca-Salas, A. Sánchez-Ferro, J. A. Obeso, “Actualización en la Enfermedad de Parkinson,” Rev. Med. Clin. Las Condes, vol. 27, no. 3, pp. 363–379, 2016.
J. Quinzaños-Fresnedo y A. I. Pérez San Pablo, “Rehabilitación del Paciente Con Enfermedad de Parkinson,” Bol. Méd. Inform. Inst. Nac. Rehab., no. 79, pp. 2–25, 2021. [En línea]. Disponible en: https://www.inr.gob.mx/Descargas/boletin/079Boletin.pdf
L. Borzì, M. Varrecchia, G. Olmo, C. A. Artusi, et al., “Home monitoring of motor fluctuations in Parkinson’s disease patients,” J. Reliable Intell. Environ., vol. 5, no. 3, pp. 145–162, 2019, doi: https://doi.org/10.1007/s40860-019-00086-x
M. Barrachina-Fernández, A. M. Maitín, C. Sánchez-Ávila, J. P. Romero, “Wearable Technology to Detect Motor Fluctuations in Parkinson’s Disease Patients: Current State and Challenges,” Sensors, vol. 21, no. 12, art. no. 4188, 2021, doi: https://doi.org/10.3390/s21124188
M. A. Hobert, W. Maetzler, K. Aminian, L. Chiari, “Technical and clinical view on ambulatory assessment in Parkinson's disease,” Acta Neurol. Scand., vol. 130, no. 3, pp. 139–147, 2014, doi: https://doi.org/10.1111/ane.12248
F. M. Rast y R. Labruyère, “Systematic review on the application of wearable inertial sensors to quantify everyday life motor activity in people with mobility impairments,” J. NeuroEngineering Rehabil., vol. 17, no. 1, art. no. 148, 2020, doi: https://doi.org/10.1186/s12984-020-00779-y
S. Jung, M. Michaud, L. Oudre, E. Dorveaux, L. Gorintin, N. Vayatis, D. Ricard, “The Use of Inertial Measurement Units for the Study of Free Living Environment Activity Assessment: A Literature Review,” Sensors, vol. 20, no. 19, art. no. 5625, 2020, doi: https://doi.org/10.3390/s20195625
A. I. Pérez Sanpablo, A. Meneses Peñaloza, J. Quinzaños Fresnedo, V. Bueyes Roiz, I. Quiñones Uriostegui, C. Hernandez Arenas, “Accuracy of discriminant analysis methods to classify activities of subjects with Parkinson’s Disease using wearable sensors,” Arch. Phys. Med. Rehab., vol. 102, no. 10, art. no. e103, 2021, doi: https://doi.org/10.1016/j.apmr.2021.07.797
H. Nguyen, K. Lebel, S. Bogard, E. Goubault, P. Boissy, C. Duval, “Using Inertial Sensors to Automatically Detect and Segment Activities of Daily Living in People With Parkinson’s Disease,” IEEE Trans. Neural Syst. Rehabil. Eng., vol. 26, no. 1, pp. 197–204, 2018, doi: https://doi.org/10.1109/TNSRE.2017.2745418
A. Rana, A. Dumka, R. Singh, M. K. Panda, N. Priyadarshi, B. Twala, “Imperative Role of Machine Learning Algorithm for Detection of Parkinson’s Disease: Review, Challenges and Recommendations,” Diagnostics, vol. 12, no. 8, art. no. 2003, 2022, doi: https://doi.org/10.3390/diagnostics12082003
M. Awais, L. Chiari, E. A. F. Ihlen, J. L. Helbostad, L. Palmerini, “Classical Machine Learning Versus Deep Learning for the Older Adults Free-Living Activity Classification,” Sensors, vol. 21, no. 14, art. no. 4669, 2021, doi: https://doi.org/10.3390/s21144669
L. R. Montero, J. A. Bastian, A. I. P. SanPablo, “Classification of Activities of Daily Living in Subjects with Parkinson’s Disease using Artificial Neural Networks,” en 2023 Global Medical Engineering Physics Exchanges/Pacific Health Care Engineering (GMEPE/PAHCE), Songdo, Korea, Republic of, 2023, pp. 1–5, doi: https://doi.org/10.1109/GMEPE/PAHCE58559.2023.10226479
N. González García y A. Taborda Londoño, “Análisis de Componentes Principales Sparse, Formulación, algoritmos e implicaciones en análisis de datos,” Tesis de maestría, Univ. Sal., Salamanca, España, 2015. [En línea]. Disponible en: https://gredos.usal.es/bitstream/handle/10366/126046/TFM_MAADM_Gonz%C3%A1lez_Taborda.pdf?sequence=4
I. T. Jollife y J. Cadima, “Principal component analysis: A review and recent developments,” Philos. Trans. A Math. Phys. Eng. Sci., vol. 374, art. no. 2065, 2016, doi: https://doi.org/10.1098/rsta.2015.0202
U. Dillmann, C. Holzhoffer, Y. Johann, S. Bechtel, et al., “Principal Component Analysis of gait in Parkinson’s disease: relevance of gait velocity,” Gait Posture, vol. 39, no. 3, pp. 882–887, 2014, doi: https://doi.org/10.1016/j.gaitpost.2013.11.021
T. Varrecchia, S. F. Castiglia, A. Ranavolo, C. Conte, et al., “An artificial neural network approach to detect presence and severity of Parkinson’s disease via gait parameters,” PLoS ONE, vol. 16, no. 2, 2021, art. no. e0244396, 2021, doi: https://doi.org/10.1371/journal.pone.0244396
A. Rana, A. S. Rawat, A. Bijalwan, H. Bahuguna, “Application of Multi Layer (Perceptron) Artificial Neural Network in the Diagnosis System: A Systematic Review,” 2018 International Conference on Research in Intelligent and Computing in Engineering (RICE), San Salvador, El Salvador, 2018, pp. 1–6, doi: https://doi.org/10.1109/RICE.2018.8509069
A. D. Pano-Azucena, E. Tlelo-Cuautle, S. X.-D. Tan, B. Ovilla-Martinez, L. G. De la Fraga, “FPGA-Based Implementation of a Multilayer Perceptron Suitable for Chaotic Time Series Prediction,” Technologies, vol. 6, no. 4, art. no. 90, 2018, doi: https://doi.org/10.3390/technologies6040090
J. Zou, Y. Han, S. S. So, “Overview of artificial neural networks,” Methods Mol. Biol., vol. 458, pp. 15–23, 2008, doi: https://doi.org/10.1007/978-1-60327-101-1_2
G. AlMahadin, A. Lotfi, M. M. Carthy, P. Breedon, “Enhanced Parkinson’s Disease Tremor Severity Classification by Combining Signal Processing with Resampling Techniques,” SN Comp. Sci., vol. 3, art. no. 63, 2022, doi: https://doi.org/10.1007/s42979-021-00953-6
L. Sigcha, B. Domínguez, L. Borzì, N. Costa, et al., “Bradykinesia Detection in Parkinson’s Disease Using Smartwatches’ Inertial Sensors and Deep Learning Methods,” Electronics, vol. 11, no. 23, art. no. 3879, 2022, doi: https://doi.org/10.3390/electronics11233879
R. LeMoyne, T. Mastroianni, D. Whiting, N. Tomycz, “Preliminary Network Centric Therapy for Machine Learning Classification of Deep Brain Stimulation Status for the Treatment of Parkinson’s Disease with a Conformal Wearable and Wireless Inertial Sensor,” Adv. Parkinson’s Dis., vol. 8, no. 4, pp. 75–91, 2019, doi: https://doi.org/10.4236/apd.2019.84007
A. Fred Agarap, “Deep Learning using Rectified Linear Units (ReLU),” 2018, arXiv: 1803.08375, doi: https://doi.org/10.48550/arXiv.1803.08375
J. Lederer, “Activation Functions in Artificial Neural Networks: A Systematic Overview,” 2021, arXiv: 2101.09957, doi: https://doi.org/10.48550/arXiv.2101.09957
J. P. Larsen, E. Dupont, E. Tandberg, “Clinical diagnosis of Parkinson’s disease. Proposal of diagnostic subgroups classified at different levels of confidence,” Acta Neurol. Scand., vol. 89, no. 4, pp. 242–251, 2009, doi: https://doi.org/10.1111/j.1600-0404.1994.tb01674.x
C. Sweeney, E. Ennis, M. Mulvenna, R. Bond, S. O’Neill, “How Machine Learning Classification Accuracy Changes in a Happiness Dataset with Different Demographic Groups,” Computers, vol. 11, no. 5, art. no. 83, 2022, doi: https://doi.org/10.3390/computers11050083
M. Greenacre, P. J. F. Groenen, T. Hastie, A. I. D’Enza, A. Markos, E. Tuzhilina, “Principal component analysis,” Nat. Rev. Methods Primers, vol. 2, art. no. 100, 2022, doi: https://doi.org/10.1038/s43586-022-00184-w
N. Japkowicz, “Assessment Metrics for Imbalanced Learning,” en Imbalanced Learning: Foundations, Algorithms, and Applications, H. He y Y. Ma, Eds., Hoboken, New Jersey, Estados Unidos: Wiley, 2013, cap. 8, pp. 187–206, doi: https://doi.org/10.1002/9781118646106.ch8
M. Bekkar, H. K. Djemaa, T. A. Alitouche, “Evaluation Measures for Models Assessment over Imbalanced Data Sets,” J. Inf. Eng. Appl., vol. 3, no. 10, pp. 27–38, 2013, [Online]. Disponible en: https://www.iiste.org/Journals/index.php/JIEA/article/view/7633/8051
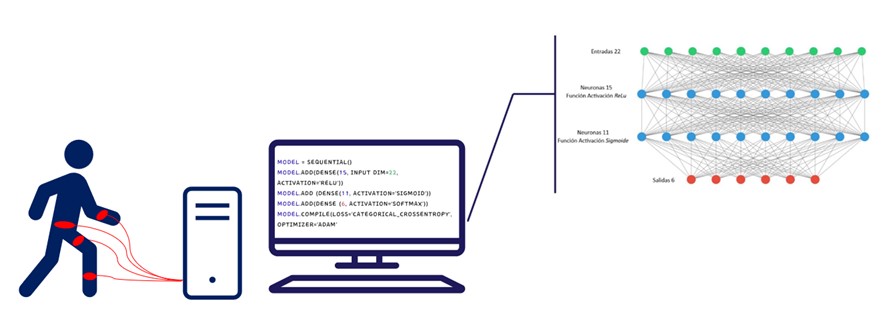
Publicado
Cómo citar
Número
Sección
Licencia
Derechos de autor 2023 Revista Mexicana de Ingenieria Biomedica

Esta obra está bajo una licencia internacional Creative Commons Atribución-NoComercial 4.0.
Una vez que el artículo es aceptado para su publicación en la RMIB, se les solicitará al autor principal o de correspondencia que revisen y firman las cartas de cesión de derechos correspondientes para llevar a cabo la autorización para la publicación del artículo. En dicho documento se autoriza a la RMIB a publicar, en cualquier medio sin limitaciones y sin ningún costo. Los autores pueden reutilizar partes del artículo en otros documentos y reproducir parte o la totalidad para su uso personal siempre que se haga referencia bibliográfica al RMIB. No obstante, todo tipo de publicación fuera de las publicaciones académicas del autor correspondiente o para otro tipo de trabajos derivados y publicados necesitaran de un permiso escrito de la RMIB.